Enhancing Supplier Performance and Risk Management with AI/ML
“Advanced AI supplier performance tools and machine learning in procurement are transforming risk management and supplier evaluation. Predictive supplier management delivers actionable insights while AI-driven analytics optimise metrics, costs, and relationships across complex supply chains, creating resilient procurement strategies.”
In today’s interconnected global economy, effective supplier performance and risk management are critical determinants of organisational success. The complexity of modern supply chains—spanning multiple tiers, geographies, and regulatory environments—presents significant challenges for procurement teams aiming to maintain visibility and control. Against this backdrop, artificial intelligence (AI) and machine learning (ML) technologies are emerging as powerful tools for transforming traditional procurement practices.
These advanced technologies offer unprecedented capabilities in data processing, pattern recognition, and predictive analysis that far exceed traditional manual methods. By implementing AI-driven supplier insights and ML supplier evaluation frameworks, organisations can process vast quantities of supplier data to identify trends, predict potential disruptions, and optimise performance metrics in ways previously unattainable.
AI procurement strategies and supplier risk analytics are reshaping the supplier management landscape, providing concrete examples of implementation and quantifiable results from industry leaders. These technologies enable more data-driven decision-making processes that enhance resilience, efficiency, and competitive advantage. For instance, predictive supplier management tools can forecast potential risks, allowing companies to mitigate issues before they escalate. Additionally, AI supplier performance metrics offer a granular view of supplier reliability and quality, facilitating more informed and strategic procurement decisions. By leveraging these AI and ML capabilities, organisations can achieve a more robust and responsive supply chain, ultimately driving better business outcomes.
Demand Forecasting
Effective demand forecasting serves as the foundation for supplier management excellence, directly impacting inventory levels, production schedules, and ultimately, financial performance. Traditional forecasting methods reliant on historical data and simple statistical models frequently fail to capture the complexity of modern market dynamics, resulting in forecast errors ranging from 25% to 40%.
Machine learning in procurement introduces sophisticated algorithms capable of synthesising multiple data streams—historical sales, economic indicators, social media trends, weather patterns, and competitor activities—to generate more accurate predictions. Deep learning models and neural networks can identify non-linear relationships and subtle patterns invisible to conventional systems.
A notable implementation comes from Tesco, which deployed ML algorithms to analyse over 200 variables affecting product demand. This AI-enhanced forecasting system reduced stockouts by 30% and overstock situations by 20%, demonstrating the tangible benefits of predictive supplier management. The system particularly excelled during high-volatility periods such as holidays and promotional events, where traditional forecasting methods typically experience the greatest deviation. [4]
The enhanced accuracy of these forecasts allows procurement teams to coordinate more effectively with suppliers, providing them with more reliable order projections and reducing the bullwhip effect throughout the supply chain.
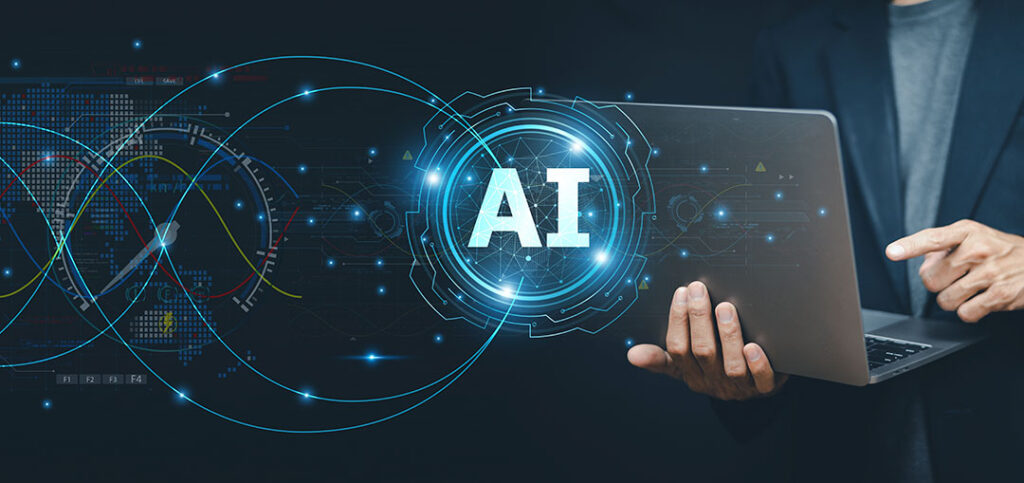
Supplier Performance and Risk Management
Comprehensive evaluation of supplier performance and associated risks represents a core procurement function that directly impacts production continuity, product quality, and operational efficiency. Traditional approaches to supplier performance monitoring often suffer from data fragmentation, delayed reporting, and limited analytical capabilities.
AI supplier performance technologies enable a fundamental shift from reactive to proactive management by continuously monitoring numerous performance indicators across multiple dimensions. Machine learning algorithms can process structured data (delivery times, quality metrics, pricing adherence) alongside unstructured data (news reports, social media sentiment, financial filings) to create comprehensive supplier risk profiles.
Natural Language Processing (NLP) applications scan regulatory updates, news articles, and social media to identify potential disruptions before they affect operations. Simultaneously, anomaly detection algorithms flag unusual patterns in supplier behaviour that might indicate potential quality issues or financial distress.
BMW’s implementation of machine learning models for predicting supplier failures achieved an 86% accuracy rate in classification tasks. Their system analyses over 30 distinct performance metrics and external risk factors to generate early warning signals, enabling procurement teams to implement mitigation strategies before disruptions occur. This proactive stance has reduced BMW’s production disruptions from supplier issues by approximately 35%. [5]
The risk management tools AI provides extend beyond operational metrics to encompass sustainability compliance, financial stability, and geopolitical exposure—creating multidimensional risk assessments that facilitate more informed decision-making.
Supplier Selection
The supplier selection process constitutes a strategic decision with long-term implications for organisational performance. Traditional selection methods often rely heavily on price considerations and subjective assessments, potentially overlooking critical risk factors and performance capabilities.
AI-driven supplier insights transform this process by enabling comprehensive evaluation across numerous weighted criteria. Machine learning algorithms can objectively analyse historical performance data, financial stability indicators, geographical risk exposure, and sustainability compliance to identify optimal supplier partnerships.
These ML supplier evaluation frameworks can process thousands of data points per potential supplier—far exceeding human analytical capabilities—to identify the candidates most aligned with organisational requirements. The technology particularly excels at detecting non-obvious relationships between supplier characteristics and performance outcomes.
Samsung Electronics implemented an AI-based supplier selection system that reduced their selection process time by 50% whilst simultaneously improving the quality of selections. Their system utilises ensemble learning techniques to evaluate suppliers across 75 distinct parameters, including traditional metrics and advanced predictive indicators of future performance. [6]
The enhanced objectivity of AI-powered selection processes also reduces the influence of cognitive biases and personal relationships that can undermine traditional supplier selection, ensuring decisions align more closely with organisational objectives and risk tolerance.
Contract Management
Effective contract management is crucial for ensuring supplier relationships deliver expected value while minimising legal and compliance risks. Traditional contract management processes, often manual and paper-based, frequently encounter issues such as version control problems, missed obligations, and limited visibility into contract performance.
AI procurement strategies have significantly improved this domain through automated contract analysis systems that utilise natural language processing to extract key terms, conditions, obligations, and risks from complex legal documents. These systems continuously monitor contractual compliance and automatically flag deviations or approaching deadlines.
Machine learning algorithms can analyse thousands of contracts simultaneously, identifying inconsistent terms, unfavourable clauses, and opportunities for standardisation or improvement across the contract portfolio. This capability is particularly valuable for large organisations managing extensive supplier agreements.
Pfizer implemented an AI-powered contract management system that reduced contract review time by 40% while enhancing compliance monitoring. Their system automatically extracts over 50 critical data points from each contract and continuously monitors adherence to terms. It has identified significant savings opportunities through the automated identification of duplicate services, missed volume discounts, and pricing inconsistencies. [7]
Beyond efficiency gains, these systems enhance risk management by ensuring greater contractual compliance and providing early warnings of potential disputes or performance issues. This proactive approach not only mitigates risks but also fosters stronger supplier relationships and ensures consistent adherence to contractual obligations.
Cost Optimisation
Strategic cost optimisation represents a perpetual objective for procurement organisations seeking to balance price considerations with quality, reliability, and risk factors. Traditional approaches to cost management often focus narrowly on unit price negotiations without considering total cost of ownership or identifying systemic inefficiencies.
AI supplier performance systems enable more sophisticated approaches to cost optimisation by analysing spending patterns across the organisation, identifying consolidation opportunities, and forecasting cost trends based on market dynamics. Machine learning algorithms can process millions of transactions to identify anomalies, duplications, and non-compliance with negotiated terms.
These systems excel at identifying non-obvious cost-saving opportunities, such as optimal order quantities, transportation consolidation possibilities, and payment timing strategies that traditional analysis might miss. Additionally, they can simulate the potential impact of different procurement strategies before implementation.
DHL implemented ML algorithms to optimise their logistics operations, resulting in a 15% reduction in transportation costs. Their system analyses historical shipment data, vehicle capacity, delivery routes, and external factors such as traffic and weather patterns to recommend optimal load consolidation strategies. The system continuously learns from new data, constantly refining its recommendations as conditions change. [8]
AI-driven cost optimisation extends beyond direct price reductions to encompass process efficiencies, working capital improvements, and risk mitigation benefits that contribute to overall value creation.
Sustainability and Compliance
Environmental, social, and governance (ESG) considerations have become increasingly critical components of supplier management strategies. Traditional compliance monitoring approaches often struggle with limited visibility into supplier practices, particularly beyond tier-one relationships.
Supplier relationship AI technologies enhance sustainability management by automating the collection and verification of compliance data across complex supply networks. Machine learning algorithms can analyse supplier sustainability reports, audit results, certification documents, and external data sources to create comprehensive compliance profiles for each supplier.
These systems excel at identifying discrepancies between reported practices and observable outcomes, flagging potential greenwashing or compliance violations for further investigation. Natural language processing capabilities enable the continuous monitoring of regulatory changes across multiple jurisdictions, ensuring procurement policies remain current.
H&M implemented an AI-driven sustainability monitoring system that improved their overall sustainability score by 25%. Their system tracks over 100 sustainability metrics across more than 750 suppliers and utilises computer vision technology to verify working conditions through factory camera feeds. This approach has significantly enhanced transparency throughout their supply chain and reduced compliance risks. [9]
The integration of supplier risk analytics with sustainability monitoring enables organisations to align procurement practices with corporate social responsibility objectives whilst minimising exposure to reputational damage and regulatory penalties.
Price Variance Analysis and Impact
Effective management of price volatility constitutes a significant challenge for procurement organisations operating in dynamic markets with fluctuating commodity prices and currency exchange rates. Traditional approaches to price variance analysis often provide limited insights into causality and future trends.
AI-driven supplier insights transform price variance analysis through advanced pattern recognition capabilities that can identify the underlying drivers of price changes. Machine learning algorithms analyse historical pricing data alongside external factors such as commodity indices, currency fluctuations, geopolitical events, and industry-specific indicators to explain observed variances and predict future trends.
These predictive supplier management systems enable procurement teams to distinguish between temporary price fluctuations and permanent structural changes, informing more strategic response strategies. Additionally, they can simulate the potential impact of different hedging strategies and contract structures on price stability.
Nestlé deployed an ML-based price variance analysis system that enabled them to negotiate better contracts and save 10% on procurement costs. Their system analyses pricing data across thousands of SKUs and hundreds of suppliers, identifying arbitrage opportunities and optimising the timing of purchase decisions. The system has proven particularly valuable for managing volatility in agricultural commodity prices. [10]
By integrating price variance analysis with broader supplier performance metrics, organisations can develop more sophisticated procurement strategies that balance price considerations with reliability, quality, and risk factors.
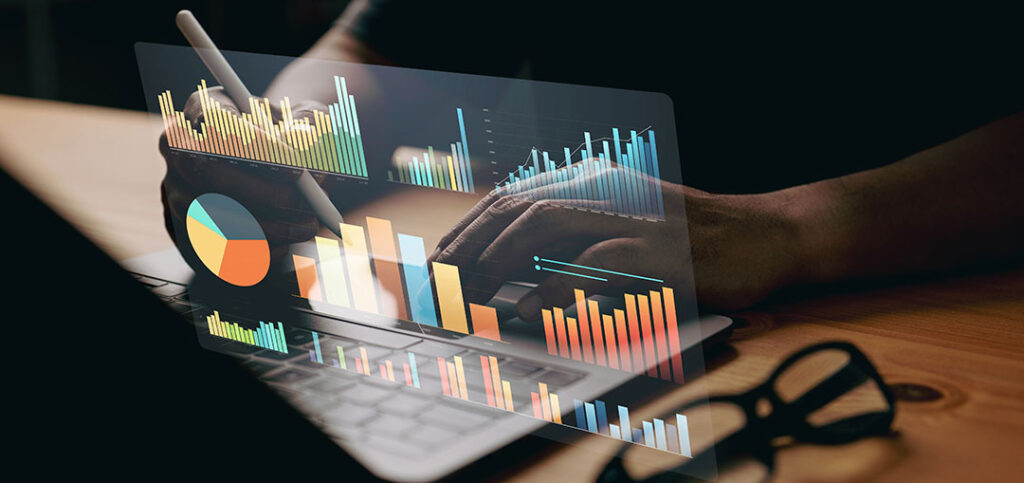
Fraud Detection
Procurement fraud poses a significant risk to organisational integrity and financial performance, with the Association of Certified Fraud Examiners estimating that companies lose approximately 5% of annual revenue to fraudulent activities. Traditional fraud detection methods often rely on periodic audits and manual reviews that may fail to detect sophisticated schemes.
Risk management tools powered by AI enable continuous monitoring of procurement transactions to identify suspicious patterns and anomalies indicative of potential fraud. Machine learning algorithms analyse purchasing data, vendor information, employee behaviours, and transaction details to detect irregular activities such as bid rigging, invoice manipulation, and conflicts of interest.
These systems excel at identifying subtle patterns that might escape human detection, such as unusual timing of transactions, relationships between supposedly independent suppliers, or statistical anomalies in pricing structures. As the algorithms continuously learn from new data, they become increasingly effective at distinguishing between legitimate variations and potentially fraudulent activities.
Unilever implemented an AI-driven fraud detection system that reduced fraudulent transactions by 35%. Their system analyses over 50 risk indicators across millions of transactions to identify potential issues for investigation. The system has proven particularly effective at detecting collusion between employees and suppliers—a notoriously difficult form of fraud to identify through traditional means. [11]
Beyond direct financial savings, these systems contribute to a culture of transparency and accountability throughout the procurement process, deterring potential fraudsters and ensuring greater adherence to ethical standards.
Conclusion
The integration of AI and ML technologies into supplier performance and risk management represents a significant transformation of procurement practices. As demonstrated through the various applications explored, these technologies enable more data-driven, proactive, and strategic approaches to supplier management that directly contribute to organisational resilience and competitive advantage.
The capabilities discussed—from enhanced demand forecasting and supplier selection to automated contract management and fraud detection—collectively enable procurement organisations to achieve unprecedented levels of visibility, control, and optimisation across their supplier networks. The tangible benefits realised by industry leaders underscore the potential value of these technologies.
Motherson Technology Services specialises in implementing these advanced technologies through customised solutions tailored to specific organisational requirements. By leveraging expertise in AI supplier performance systems and machine learning in procurement, organisations can accelerate their digital transformation journeys and establish more resilient, efficient, and sustainable supplier relationships.
As market volatility and supply chain complexity continue to increase, the adoption of AI-driven supplier insights and ML supplier evaluation frameworks will increasingly differentiate procurement leaders from laggards. Organisations that establish the necessary technological infrastructure and analytical capabilities today will be best positioned to navigate the challenges of tomorrow’s supply landscape.
References
[2] https://actalogistica.eu/issues/2024/III_2024_05_Rezki_Mansouri.pdf
[6] https://www.samsung.com/global/sustainability/popup/popup_doc/AYUqtdzKDlwAIx_C/
[7] https://cdn.pfizer.com/pfizercom/AI_Policy_Position_12112023.pdf
[9] https://hmgroup.com/our-stories/responsible-ai-is-better-ai/
[10] https://digitaldefynd.com/IQ/nestle-using-ai/
[11] https://www.unite.ai/how-ai-agents-are-reshaping-security-and-fraud-detection-in-the-business-world/
About the Author:
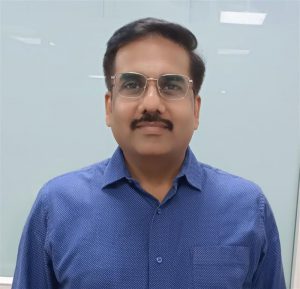
Arvind Mishra, Associate Vice President & Head, Digital and Analytics, Motherson Technology Services. A strong leader and technology expert, he has nearly 2 decades of experience in the technology industry with specialties in data-driven digital transformation, algorithms, Design and Architecture, and BI and analytics. Over these years, he has worked closely with global clients in their digital and data/analytics transformation journeys across multiple industries.