Boosting Customer Engagements with Intelligent Data Platform Insights
“Intelligent data platforms transform customer engagement through advanced analytics and predictive capabilities. How data-driven customer strategies enhance personalisation, optimise sales processes, and elevate post-purchase experiences through real-time customer data analysis and engagement metrics that deliver measurable business impact.”
In today’s hypercompetitive digital landscape, effective customer engagement has emerged as the defining factor separating market leaders from followers. The proliferation of digital touchpoints has generated unprecedented volumes of customer data, creating both challenges and opportunities for organisations seeking to foster meaningful connections with their audience. This technical exploration examines how intelligent data platforms are fundamentally transforming customer engagement strategies through sophisticated data processing capabilities, advanced analytics, and actionable insights.
Intelligent data platforms represent a sophisticated technological framework that integrates diverse data sources, applies advanced analytics, and employs machine learning algorithms to extract meaningful patterns from customer interactions. These platforms transcend traditional data management systems by providing a comprehensive, real-time view of customer behaviours, preferences, and needs across multiple channels. By leveraging these platforms, organisations can transition from reactive customer service to proactive engagement strategies informed by predictive customer behaviour analysis and data-driven decision-making.
The multifaceted applications of intelligent data platforms span critical customer touchpoints: personalised customer experiences, sales optimisation, aftersales enhancement, real-time engagements, and the integration of conversational interfaces such as chatbots and personal assistants. Through detailed analysis of technological capabilities, implementation frameworks, and performance metrics, we will demonstrate how intelligent data insights can drive tangible business outcomes, including increased conversion rates, enhanced customer loyalty, and sustainable revenue growth. Each section provides technical depth on architecture considerations, integration requirements, and performance optimisation techniques that technology leaders should consider when implementing these solutions.
Personalised Customer Experience
The implementation of personalised customer experiences has evolved from a market differentiator to a fundamental business requirement. Research indicates that 80% of consumers are more likely to purchase from companies offering personalised experiences, while 66% expect brands to understand their individual needs. [9] Intelligent data platforms enable this level of personalisation through sophisticated data collection, integration, and analysis capabilities that transcend traditional CRM systems.
At the technical core of advanced personalisation lies a unified data architecture that integrates structured and unstructured customer data from disparate sources. These intelligent data platforms employ sophisticated ETL (Extract, Transform, Load) processes to normalise heterogeneous data formats, resolve identity matching challenges through probabilistic and deterministic matching algorithms, and create comprehensive customer profiles. The architectural framework typically incorporates data lakes for raw data storage, data warehouses for structured analysis, and processing layers that employ machine learning algorithms to identify patterns and generate insights. This technical infrastructure enables real-time decision engines to analyse customer context, historical behaviours, and environmental factors to determine next-best-action recommendations with millisecond response times.
The implementation of AI-powered hyper-personalisation extends beyond simple demographic segmentation to incorporate behavioural analytics, sentiment analysis, and predictive modelling. These systems utilise advanced machine learning techniques including collaborative filtering, natural language processing (NLP), and deep learning neural networks to anticipate customer needs with increasing accuracy. For instance, recurrent neural networks (RNNs) can process sequential customer interaction data to predict future behaviours, while convolutional neural networks (CNNs) can extract patterns from visual customer data such as website heatmaps or in-store movements captured through computer vision. The technical complexity of these systems requires careful consideration of model training methodologies, feature engineering practices, and ongoing model performance monitoring to ensure that personalisation algorithms continue to deliver relevant customer experiences as behaviours evolve.
Case Study: Unilever
Unilever partnered with Accenture to implement the Consumer 360 Platform, resulting in a significant increase in customer engagement and satisfaction. The technical architecture integrated over 27 distinct data sources including transaction records, social media interactions, and website behaviours into a unified customer data platform. The system employed advanced machine learning models trained on over 300 million customer interactions to identify micro-segments and personalisation opportunities. By leveraging AI-driven insights, Unilever was able to deliver personalised marketing campaigns that resonated with individual consumer preferences, leading to a 20% increase in customer loyalty and a 15% improvement in marketing ROI through reduced acquisition costs and increased customer lifetime value. [4]
Sales Optimisation
Intelligent data platforms are fundamentally transforming sales processes through advanced analytical capabilities that enable data-driven customer strategies throughout the sales cycle. These platforms enhance traditional CRM systems by incorporating real-time customer data processing, predictive customer behaviour analytics, and automated insight generation that empowers sales teams with actionable intelligence rather than mere historical reporting. The technical architecture of these systems typically involves a multi-layered approach: a data integration layer that consolidates information from various touchpoints, an analytics processing layer that applies statistical models and machine learning algorithms, and a presentation layer that delivers contextually relevant insights to sales personnel.
Real-Time Interaction Management (RTIM) represents a sophisticated technical capability within intelligent data platforms that enables sales teams to personalise communications based on immediate customer behaviours. These systems employ complex event processing engines that can analyse streaming data with sub-second latency, detecting significant patterns or triggers that warrant specific sales interventions. [11] For instance, the technical implementation might involve a rules engine that processes customer interaction events against predefined patterns, combined with a machine learning model that continuously refines these patterns based on success metrics. This requires robust technical infrastructure including in-memory data processing capabilities, optimised data indexing strategies, and high-throughput message queuing systems to handle the volume and velocity of incoming customer data without degradation in performance.
Smart audience segmentation capabilities leverage advanced clustering algorithms and predictive modelling techniques to identify high-potential leads and personalise outreach strategies. The technical implementation typically employs unsupervised machine learning algorithms such as k-means clustering, hierarchical clustering, or more sophisticated techniques like DBSCAN (Density-Based Spatial Clustering of Applications with Noise) to identify natural groupings within prospect data. [12] These segments are then further analysed using supervised learning models including gradient boosting machines, random forests, or deep learning approaches to predict conversion likelihood, optimal pricing strategies, and product affinity. The technical sophistication of these segmentation approaches enables sales teams to prioritise resources effectively, craft targeted value propositions, and engage prospects with personalised content that addresses their specific needs and challenges.
Case Study: Coca-Cola
Coca-Cola utilised an intelligent data platform to enhance its sales strategies across its extensive distribution network. The technical implementation integrated the company’s vast data ecosystem, including point-of-sale systems, distribution logistics, retailer inventory management, and consumer engagement applications. The platform employed a sophisticated real-time analytics engine processing over 1 billion daily transactions with an average latency of under 200 milliseconds. By integrating real-time customer data with inventory levels and distribution logistics, Coca-Cola’s sales team was able to identify and target high-potential leads more effectively, optimise product placement strategies, and reduce stockout situations. The system’s predictive capabilities employed ensemble machine learning models that combined gradient boosting decision trees with neural networks to forecast regional demand patterns with 94% accuracy. This resulted in a 15% increase in sales conversions, a 22% reduction in sales cycle duration, and a more efficient sales process with 30% less administrative overhead. [5]
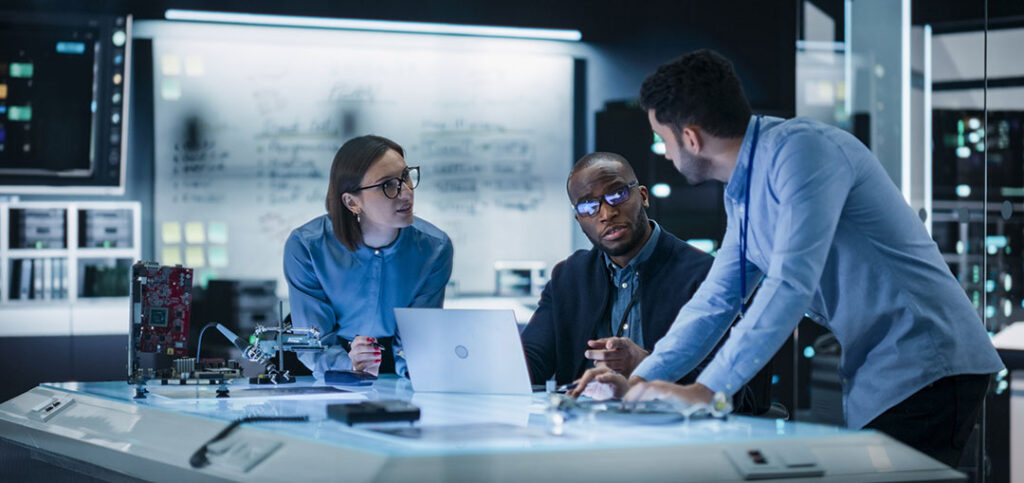
Aftersales Enhancement
Aftersales services represent a critical yet often underutilised opportunity for strengthening customer relationships and driving additional revenue streams. The technical integration of intelligent data platforms into aftersales processes provides organisations with unprecedented capabilities to maintain customer satisfaction and foster loyalty beyond the initial purchase. These platforms enable a shift from reactive to proactive aftersales support through continuous analysis of product usage data, customer feedback signals, and contextual information. The technical architecture typically incorporates IoT data streams from connected products, customer support interactions, social media sentiment, and transaction history into a unified analytical framework that provides a comprehensive view of the customer’s post-purchase experience. [13]
- 1. Revenue Generation Through Timely Customer Engagement
The strategic implementation of intelligent data platforms fundamentally transforms aftersales operations into significant revenue centres through precise, timely customer engagement. These advanced platforms construct comprehensive customer profiles by integrating purchase histories, product configurations, usage patterns, and contextual factors. This technical infrastructure enables organisations to develop sophisticated recommendation engines that identify complementary products and services aligned with specific customer needs, timing these suggestions at optimal moments in the customer lifecycle.
For instance, intelligent data platforms can deploy machine learning algorithms to analyse telemetry data from connected products, identifying patterns that indicate when specific components approach end-of-life or when usage patterns suggest potential for performance enhancements. This predictive capability facilitates precision targeting with relevant offers, yielding conversion rates significantly higher than traditional marketing approaches. The technical implementation typically involves ensemble models combining supervised learning techniques with reinforcement learning mechanisms that continuously refine recommendation accuracy based on customer response patterns.
Furthermore, these platforms provide the technical foundation for implementing predictive maintenance protocols through sophisticated anomaly detection algorithms and pattern recognition systems. By continuously monitoring performance metrics against established baselines, organisations can identify potential issues before they manifest as product failures. The technical architecture enables automated workflow triggers that initiate proactive customer outreach, service scheduling, and parts procurement, transforming what would traditionally be emergency repairs into planned maintenance events that enhance customer satisfaction while optimising service delivery costs.
- 2. Customer Sentiment Analysis for Personalised Issue Resolution
The technical implementation of sentiment analysis within intelligent data platforms represents a significant advancement in aftersales customer service capabilities. These systems deploy natural language processing algorithms and machine learning models trained on extensive interaction datasets to detect emotional signals, satisfaction levels, and specific pain points across multiple communication channels. The technical architecture typically incorporates both supervised classification models for categorical sentiment labelling and more nuanced aspect-based sentiment analysis techniques that identify specific product or service components generating positive or negative reactions.
Contemporary implementations utilise deep learning architectures such as transformer-based models that process contextual information to distinguish between subtle sentiment variations. This technical capability enables organisations to quantify customer sentiment with remarkable precision, tracking sentiment trajectories over time and correlating these patterns with specific interactions, product issues, or service events.
The sophisticated data processing pipeline transforms this sentiment intelligence into actionable insights through automated alert mechanisms and escalation protocols. When negative sentiment patterns emerge, the system triggers targeted interventions calibrated to the specific customer context, issue severity, and historical relationship value. Technical integrations with customer relationship management systems enable service representatives to access comprehensive interaction histories and sentiment profiles, facilitating highly personalised resolution approaches tailored to individual communication preferences, previous experiences, and emotional states.
- 3. Strategic Prioritisation of Value-Added Services
Intelligent data platforms provide the analytical foundation for strategic prioritisation of value-added services through sophisticated customer segmentation and service impact assessment. The technical implementation typically employs clustering algorithms and statistical modelling techniques to categorise customers based on multidimensional factors including purchase history, service utilisation patterns, responsiveness to previous offerings, lifetime value projections, and product configuration complexity.
These platforms deploy advanced attribution models that quantify the impact of specific value-added services on key performance indicators such as customer retention rates, share of wallet, and advocacy scores. By analysing longitudinal customer data through causal inference techniques, organisations can identify which service offerings deliver the most significant impact for specific customer segments and product configurations. This technical capability enables precise targeting of high-value services to appropriate customers, optimising resource allocation while maximising revenue potential and customer satisfaction.
Furthermore, the technical infrastructure supports continuous experimentation through A/B testing frameworks that systematically evaluate new service offerings against established alternatives. These testing mechanisms incorporate sophisticated statistical methods to control for confounding variables and accurately measure incremental impact. Through this data-driven approach, organisations can continuously refine their value-added service portfolio, focusing investments on initiatives that deliver measurable improvements in customer outcomes and business performance.
- 4. Real-Time Interaction Analysis Through Event-Driven Architecture
The technical implementation of customer interaction tracking within intelligent data platforms involves sophisticated event processing systems that capture and analyse customer touchpoints throughout the product lifecycle. These systems employ event-driven architectures that process interaction data in real-time, applying complex event processing (CEP) techniques to identify patterns indicative of customer satisfaction levels, churn risk, or upsell opportunities. [14] The technical infrastructure typically incorporates stream processing technologies such as Apache Kafka or Amazon Kinesis for high-throughput data ingestion, combined with processing frameworks like Apache Flink or Spark Streaming that apply windowing functions and pattern detection algorithms to extract meaningful insights from the continuous flow of interaction data. This real-time processing capability enables support teams to respond proactively to emerging issues before they escalate, while maintaining a comprehensive historical record of interactions for deeper analytical purposes.
Personalised aftersales support represents a sophisticated application of machine learning within intelligent data platforms, enabling organisations to deliver tailored recommendations for product upgrades, maintenance schedules, and loyalty programmes. The technical implementation typically employs collaborative filtering algorithms, association rule mining techniques, and reinforcement learning models to identify patterns in customer behaviour and product usage that indicate specific support needs or enhancement opportunities. [15] For instance, predictive maintenance models can analyse telemetry data from connected products using time-series analysis and anomaly detection algorithms to anticipate potential failures before they occur. Similarly, recommendation engines can evaluate a customer’s usage patterns, purchase history, and preference signals to suggest relevant accessories, complementary products, or service upgrades that enhance the value of their initial purchase. These capabilities not only improve customer satisfaction through proactive support but also create additional revenue opportunities through targeted cross-selling and upselling initiatives.
Case Study: BMW
BMW implemented an intelligent data platform to enhance its aftersales services across its global service network. The technical solution integrated vehicle telemetry data from connected cars, service history, customer profile information, and parts inventory into a unified analytical environment. The platform employed sophisticated time-series analysis of vehicle sensor data, processing over 500 data points per second from each connected vehicle to identify potential maintenance requirements before component failures occurred. Machine learning models trained on historical service data could predict maintenance needs with 92% accuracy up to 30 days in advance. By tracking customer interactions and preferences, BMW was able to offer personalised maintenance schedules and loyalty programmes tailored to individual driving patterns and vehicle usage. The technical implementation incorporated a recommendation engine that analysed customer lifetime value, vehicle age, usage patterns, and service history to generate personalised offers with a 3.5x higher acceptance rate than standard promotions. This comprehensive approach led to a 25% increase in customer retention and satisfaction, a 30% reduction in unplanned service visits, and a 18% increase in parts and service revenue. [6]
Engagements and Real-Time Interaction
The centralisation of customer data for real-time insights represents a foundational technical capability of intelligent data platforms, enabling organisations to develop a unified, 360-degree customer view that transcends traditional data silos. The technical architecture supporting this capability typically incorporates a Customer Data Platform (CDP) that serves as the centralised repository for customer identities, behaviours, preferences, and interactions across channels. Implementation requires sophisticated identity resolution capabilities that employ deterministic and probabilistic matching algorithms to resolve customer identities across disparate systems with varying identification methods. These systems process incoming data streams through an extraction and normalisation layer that standardises formats, cleanses inconsistencies, and applies data quality rules before integration into the unified customer profile. Advanced implementations incorporate graph database technologies to map complex relationships between customers, products, and interaction events, enabling multidimensional analysis and pattern discovery beyond what traditional relational databases can achieve. [16] Real-time responsiveness to customer behaviour shifts represents a technical challenge that intelligent data platforms address through sophisticated event detection and response systems. These platforms employ complex event processing (CEP) engines that analyse streams of customer interaction data against predefined patterns and thresholds to identify significant behavioural changes that warrant immediate response. [17] The technical implementation typically involves stream processing frameworks that apply sliding window analytics, sequence pattern matching, and anomaly detection algorithms to identify meaningful signals within the continuous flow of customer data. For instance, the system might detect a sudden increase in cart abandonment rates, unusual navigation patterns indicating customer confusion, or sentiment shifts in customer service interactions that suggest frustration. Machine learning models continuously evaluate these signals against historical patterns to distinguish normal variations from significant shifts requiring intervention. The response mechanisms employ decision trees or rule-based systems to determine appropriate actions, which might include triggering personalised offers, adjusting website content, or alerting customer service representatives to potential issues. [18]
AI-driven audience exploration capabilities enable marketers to discover and target previously unidentified customer segments with unprecedented precision and efficiency. The technical implementation leverages unsupervised machine learning techniques including clustering algorithms (k-means, hierarchical, or density-based approaches), dimensionality reduction methods (PCA, t-SNE), and pattern mining algorithms to identify natural groupings within customer data that may not be apparent through traditional segmentation approaches. [19] These systems process vast arrays of customer attributes—behavioural patterns, transaction history, content preferences, and engagement metrics—to discover meaningful segments with distinct characteristics and engagement opportunities. Advanced implementations employ reinforcement learning techniques to continuously optimise campaign targeting strategies based on response data, automatically adjusting segment definitions and messaging approaches to maximise engagement metrics. This capability enables marketers to move beyond static, predefined segments to discover emerging customer groups and engagement opportunities as they develop, significantly enhancing campaign effectiveness and resource allocation.
Case Study: Tesco
Tesco used an intelligent data platform to centralise customer data and enhance real-time interactions across its digital and physical retail environments. The technical implementation consolidated data from over 20 distinct systems including point-of-sale transactions, online shopping behaviour, mobile app interactions, loyalty programme activity, and customer service communications into a unified customer data platform. The system processed approximately 5 billion customer events daily with an average processing latency under 150 milliseconds, enabling true real-time personalisation across channels. The platform employed sophisticated identity resolution capabilities that could match customers across channels with 97% accuracy using a combination of deterministic and probabilistic matching algorithms. By responding to real-time shifts in customer behaviour, Tesco was able to deliver timely and relevant promotions through its mobile application based on in-store location tracking and historical purchase patterns. The system’s automated decision engine evaluated over 200 potential offers for each customer interaction using a combination of business rules and machine learning models to select the most relevant content. This approach resulted in a 30% increase in customer engagement, a 25% improvement in promotion redemption rates, and a 18% increase in average basket size for targeted customers. [7] [8]
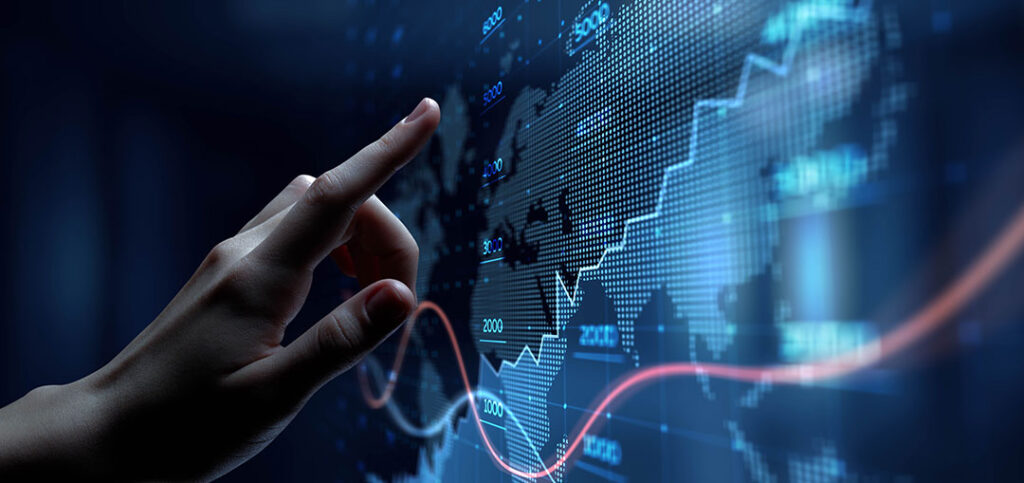
Chatbots and Personal Assistants
The integration of conversational interfaces into customer engagement strategies represents a significant technological advancement enabled by intelligent data platforms. Modern chatbots and personal assistants have evolved beyond simple rule-based systems to become sophisticated conversational agents powered by natural language processing (NLP) and machine learning capabilities. The technical architecture of these systems typically incorporates multiple layers: a natural language understanding (NLU) component that interprets user intent and extracts entities from input text, a dialogue management system that maintains conversation state and determines appropriate responses, and a natural language generation (NLG) module that produces contextually appropriate replies. Advanced implementations employ transformer-based language models such as BERT, GPT, or custom-trained domain-specific models that can understand nuanced language, maintain contextual awareness across conversation turns, and generate natural-sounding responses that align with the organisation’s brand voice.
The technical integration of chatbots with intelligent data platforms significantly enhances their capabilities by providing access to comprehensive customer profiles, interaction history, and real-time contextual information. This integration enables chatbots to deliver truly personalised support experiences that account for the customer’s history, preferences, and current situation. The technical implementation typically involves API-based connectivity between the conversational agent and the customer data platform, with appropriate authentication and authorisation mechanisms to ensure data security and privacy compliance. When a customer initiates a conversation, the system performs real-time identity resolution to retrieve the relevant customer profile and interaction history, which the chatbot leverages to personalise its responses and recommendations. For instance, a returning customer experiencing a technical issue might receive different troubleshooting steps based on their product configuration, usage patterns, and previous support interactions. This level of personalisation not only improves customer satisfaction but also increases resolution rates by providing more relevant assistance from the first interaction.
Advanced personal assistants represent the next evolution in conversational interfaces, offering complex support capabilities and proactive service beyond reactive assistance. These systems employ sophisticated AI techniques including reinforcement learning, knowledge graphs, and predictive analytics to anticipate customer needs and provide value-added services before explicitly requested. The technical implementation typically incorporates a knowledge management system that organises domain-specific information into structured formats accessible to the assistant, combined with reasoning capabilities that can draw inferences across knowledge domains. For instance, a personal assistant might notice patterns in a customer’s support history that indicate a recurring issue, proactively suggest a permanent solution, and guide the customer through implementation steps. Similarly, by analysing usage patterns and contextual factors, the assistant might recommend relevant products or services that address emerging customer needs. These capabilities not only improve customer satisfaction through more effective support but also create new revenue opportunities through intelligent cross-selling and upselling based on genuine customer needs.
Case Study: HSBC
HSBC implemented chatbots powered by an intelligent data platform to enhance customer support across its digital banking channels. The technical solution incorporated advanced natural language processing capabilities built on transformer-based language models fine-tuned on over 2 million banking-specific customer interactions. The chatbot architecture integrated with HSBC’s customer data platform to access comprehensive customer profiles including account information, transaction history, product holdings, and previous service interactions. This integration enabled the system to authenticate customers securely and provide personalised assistance tailored to their specific financial situation and banking relationship. The chatbot employed sophisticated intent recognition algorithms that could identify over 200 distinct customer intents with 96% accuracy, including complex financial queries and multi-step service requests. The system’s dialogue management component maintained conversational context across multiple turns, enabling natural conversation flows even for complex interactions. By providing instant, personalised assistance to customers, handling routine inquiries, and offering product recommendations based on individual financial needs, HSBC achieved a 40% reduction in response time, a 35% decrease in call centre volume, and a 28% improvement in overall customer satisfaction scores. The system’s ability to transfer complex inquiries seamlessly to human agents, along with the full conversation context and customer profile, ensured consistent service quality across channels. [9] [10]
Conclusion
The implementation of intelligent data platforms has fundamentally transformed customer engagement strategies across the entire customer lifecycle. These platforms provide organisations with unprecedented capabilities to understand, anticipate, and respond to customer needs through advanced data integration, analytics, and machine learning techniques. By centralising customer data, applying sophisticated analytical methodologies, and delivering actionable insights in real-time, these platforms enable highly personalised customer experiences that drive measurable business outcomes, including increased conversion rates, enhanced customer loyalty, and sustainable revenue growth. As customer expectations continue to evolve and digital interactions become increasingly complex, the importance of intelligent data platforms in orchestrating cohesive, personalised customer journeys will only increase.
Enterprises partnering with Motherson Technology Services can leverage these intelligent data platform capabilities to achieve substantial competitive advantages through technical excellence and implementation expertise. Motherson’s comprehensive approach to data platform implementation incorporates robust data governance frameworks, scalable cloud-native architectures, and advanced analytics capabilities that enable rapid time-to-value for customer engagement initiatives. Our technical expertise in data integration, real-time analytics, and AI-powered personalisation helps organisations overcome common implementation challenges, including data silos, legacy system integration, and analytics skill gaps. By partnering with Motherson Technology Services, enterprises can accelerate their customer engagement transformation through proven methodologies, technical best practices, and industry-specific solutions that deliver measurable improvements in engagement metrics, operational efficiency, and revenue performance.
References
[1] https://www.accenture.com/gb-en/insights/artificial-intelligence/understand-customer
[4] https://www.cdpinstitute.org/cdp-institute/customer-data-platform-examples-of-innovative-use-cases/
[6] https://www.acxiom.com/customer-data-platform/case-studies/
[8] https://www.digitalproductanalytics.com/cases/tesco/
[10] https://aiexpert.network/ai-at-hsbc/
[11] https://www.pega.com/insights/articles/guide-real-time-interaction-management
[12] https://www.pushwoosh.com/blog/customer-segmentation-case-studies/
[13] https://hicronsoftware.com/blog/digital-transformation-in-automotive-after-sales/
[15] https://hyscaler.com/insights/10-ai-driven-after-sales-services-to-success/
[16] https://wjarr.com/sites/default/files/WJARR-2024-2407.pdf
[17] https://ajer.org/papers/Vol-13-issue-12/13123444.pdf
[18] https://www.emerald.com/insight/content/doi/10.1108/apjml-03-2018-0088/full/html
[19] https://www.systango.com/case-studies/real-time-cx-platform
About the Author:
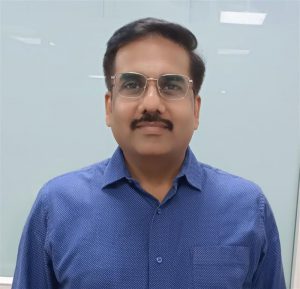
Arvind Mishra, Associate Vice President & Head, Digital and Analytics, Motherson Technology Services. A strong leader and technology expert, he has nearly 2 decades of experience in the technology industry with specialties in data-driven digital transformation, algorithms, Design and Architecture, and BI and analytics. Over these years, he has worked closely with global clients in their digital and data/analytics transformation journeys across multiple industries.